New research examines the fracture mechanisms of graphene
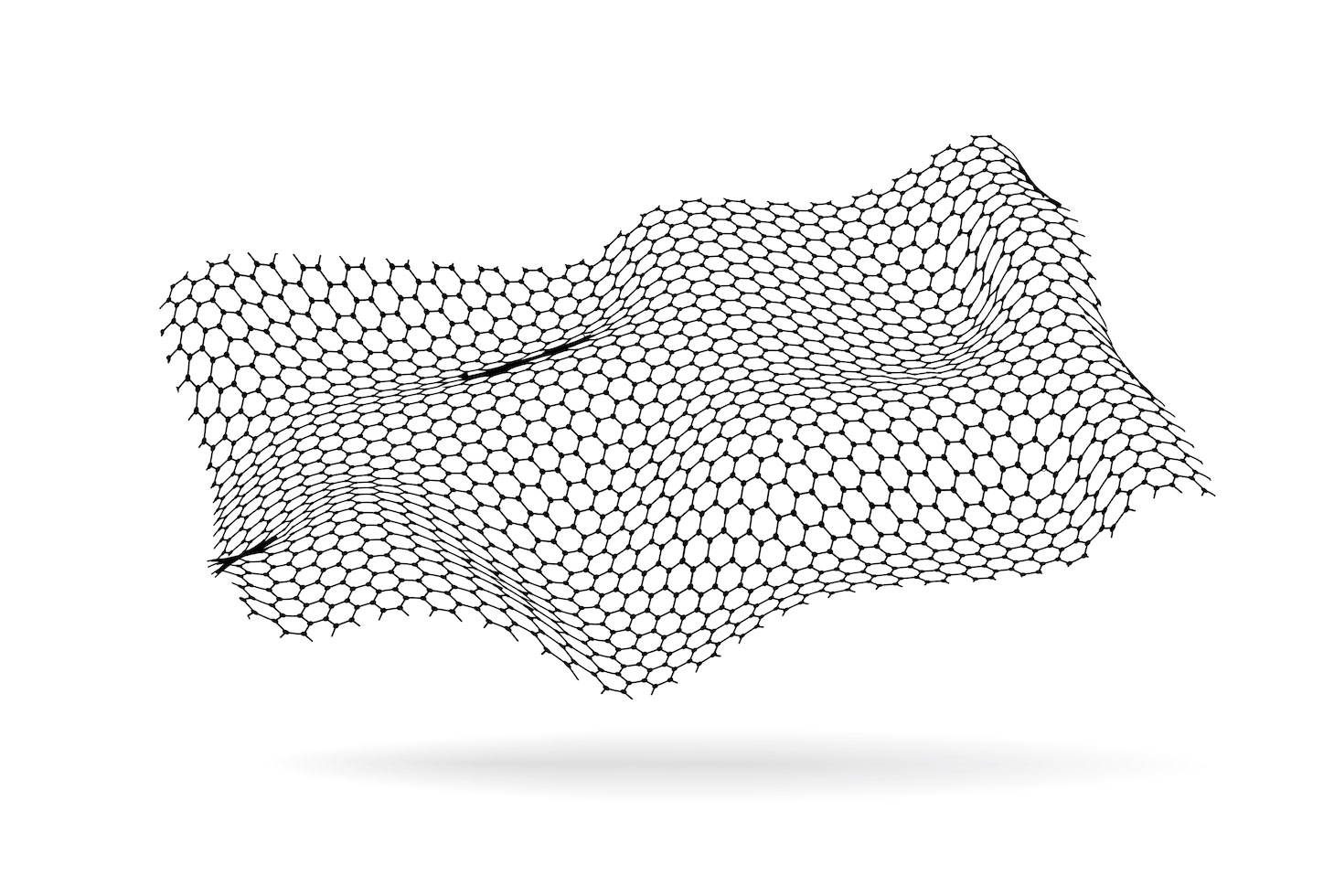
Understanding how materials fracture and break is critical to the design of resilient nanomaterials. Molecular dynamics offers a way to study fractures but is computationally expensive with limitations of scalability. To explore an alternative method, Professor Markus Buehler’s Laboratory for Atomistic and Molecular Mechanics (LAMM) group uses a machine learning model to predict the fracture evolution of graphene, the strongest material in the world. The results of the ML model, published today in npj 2D Materials and Applications, quantitatively captures how graphene fractures, including predicting the path of breakage. The research led by graduate student, Andrew Lew, provides promise toward the wider application of deep learning to materials design, opening the potential for other 2D materials. Read more about the ML model and research, “Deep learning model to predict fracture mechanisms of graphene” in npj 2D Materials and Applications.